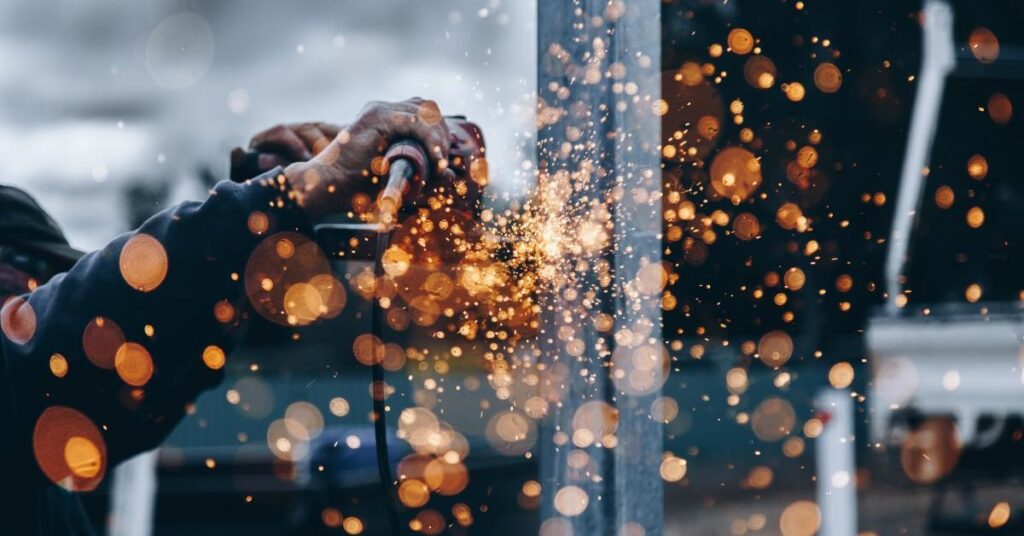
Jobs in Industrial Engineering
Industrial engineers use fundamental skills transferable to systems and management [...]
Anyone spending time on social media platforms these days has likely encountered a video of a celebrity or politician that appears real… and yet, something seems off. Frequently what they’re seeing and hearing is a fiction—they’ve encountered a deepfake (a term coined by combining deep learning—a form of machine learning—and fake). Artificial intelligence (AI) is now so sophisticated that it is used to manipulate video and audio files to make anyone appear to say or do whatever someone else wants.
So far, deepfakes are relatively easy to spot and this AI technology is mostly used to create synthetic media, such as parodies of Seinfeld and Better Call Saul or to spoof celebrities like Snoop Dogg, or Robert Downey, Jr, George Lucas, Ewan McGregor, and Jeff Goldblum (all of which is generally legal).
But there’s no question that as deepfake technology becomes more advanced and realistic, its potential for misuse by malign actors increases significantly. For instance, at the start of Russia’s invasion of Ukraine, someone posted a crude deepfake video of Ukrainian President Volodymyr Zelensky falsely telling his soldiers to lay down their weapons and not fight the Russian invaders.
The US Department of Homeland Security (DHS) has issued the publication “Increasing Threat of DeepFake Identities” to inform both the public and private sectors of deepfake’s myriad threat risks. These include bad actors weaponizing deepfake tech to interfere with elections, incite violence, produce false evidence, fake kidnappings, sabotage corporations, manipulate stocks, cyberbully marginalized groups or individuals, create deepfake pornography (putting a famous movie actress’s face on an adult film actress’s body), or prey on children (all of which, of course, is illegal).
Unfortunately, easily accessible software tools such as FakeApp and DeepFaceLab make deepfake technology available to anyone with a connection to the internet.
So, what is being done to detect and combat malign deepfakes? This article explains the process of __detecting deepfakes using data analytics and addresses:
Deepfakes rely on face recognition technology. Snapchat users already are familiar with the face swap and filter tools that alter or enhance one’s facial features. Deepfakes work similarly but are considerably more sophisticated and realistic. Artificial intelligence (AI) and machine learning methods, collectively referred to as generative adversarial networks (GANs), are used to produce these fake videos and images.A GAN can analyze thousands of pictures of the pop singer Adele and create a brand-new image that resembles those photos but isn’t a replica of any of them.
University and Program Name | Learn More |
Pepperdine University:
Online Master of Business Administration
|
|
Boston College:
Master of Science in Applied Economics
|
|
Boston College:
Master of Science in Applied Analytics
|
|
Merrimack College:
Master of Science in Data Science
|
Researchers and businesses use detection algorithm tools like Recurrent Neural Network (RNN), Convolutional Neural Network (CNN), and Long Short-term Memory (LSTM) to identify deepfakes, which frequently employ AI and deep learning. They also apply deepfake video detection models like Biological Singles Analysis and Spatial and Temporal Features Analysis to examine videos for digital abnormalities or features, such as blinking or facial tics, that deepfakes cannot duplicate accurately.
Although researchers and tech businesses have released data sets to enhance training detection technologies, these sets may be insufficient on their own. To keep deepfake detection models from losing their ability to recognize manipulated media, they must be updated regularly with more sophisticated open-source data. State-of-the-art tools cannot consistently detect deepfakes in a comprehensive, automated manner. However, there are now research programs developing ways to automatically identify deepfakes, reveal how they were made, and evaluate the overall integrity of digital content.
The DeepFake Detection Challenge (DFDC), a competition created by AWS, Microsoft, Facebook, the Partnership on AI, and academicians, is one example of a deepfake dataset technology initiative. Managed by Kaggle, DFDC offered a $1 million prize to researchers who can develop cutting-edge technologies to help detect deepfakes and manipulated media. More than 2,000 people participated, producing over 35,000 deepfake detector models.
Similarly, the MIT research project Detect Fakes seeks to identify ways to combat AI-generated misinformation. (Their site includes a test you can take to see if you can sort the deepfakes from the real videos.)
Researchers from Stanford and UC Berkeley developed an AI-driven method for detecting lip-sync technology, which reveals 80 percent of fakes by recognizing the misalignment between the shapes of people’s mouths and the sounds they produce when they speak.
While the technology for detecting deepfakes continues to improve, it may not be enough to stop a false video from spreading misinformation. Unfortunately, many viewers are unaware of deepfakes or won’t take the time to verify the integrity of the videos they’re seeing online. There is proposed legislation to launch an anti-deepfake federal taskforce; critics argue that Congress should focus more on the less-advanced but wildly effective methods of disinformation currently in use.
Photographic images have been manipulated for propagandistic reasons practically since the invention of the medium. Well-known wartime scenes from the Civil War through World War II were staged or fabricated.
More recent examples of widely-recognized deepfakes include:
Deepfake technology also is being used by the creative industries for entertainment purposes, from inserting and animating a deceased actor in a movie to making an older actor appear much younger (“de-aging”) to addressing the “uncanny valley” in the facial expressions of computer-generated characters in video games. Even business training films and physician training videos are produced using deepfake technology.
The threat from deepfakes is only expected to worsen in the future. Fortunately, it appears that data analytics offers several potentially effective solutions to combating deepfakes. One anti-deepfake strategy is to use data analytics to “poison” the intended training data by disrupting the deepfake synthesis model training procedure. Other data analytic-based methods include enlisting and further developing technologies that verify original videos via invisible digital watermarking or control capture.
Many new deepfake detection and elimination technologies originate in academia, particularly university computer science departments. Their deep-well of talented computer scientists continues to refine the use of data analytics to counteract advances in deepfake technology. Anyone intrigued by the thought of joining the good guys battling the deepfakers should look into earning a Master of Science in Data Analytics.
(Last Updated on February 26, 2024)
Questions or feedback? Email editor@noodle.com
Industrial engineers use fundamental skills transferable to systems and management [...]
IT professionals are coming out of the woodwork to secure [...]
Many people pursue their master's in industrial engineering to advance [...]
A Master of Science in Industrial Engineering provides expert knowledge [...]
Categorized as: Business Intelligence & Analytics, Data Science, Information Technology & Engineering