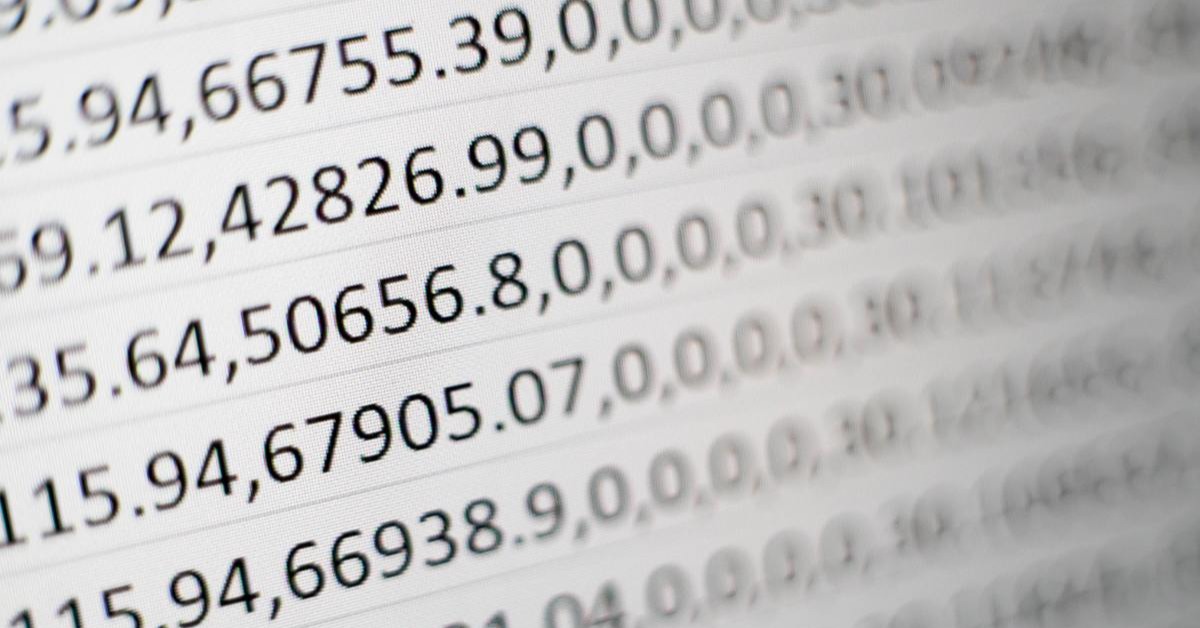
How to Become a Statistician
Learning statistical analysis, statistical methods, and statistical theory is a [...]
Knowing where technology is going to go next involves a lot of moving parts and a deep understanding of both big data and what big business requires from it. How do we know where to invest time and money in this rapidly changing work climate?
The information technology (IT) research and consultancy company Gartner has developed an interesting and useful model to interpret technology hype by measuring the viability of current and emerging technologies. Companies invest heavily in data analytics and business intelligence for data analysis and problem-solving, and Gartner’s model—The Hype Cycle—helps with decision-making for expensive and potentially risky tech investments.
The Hype Cycle outlines five key phases to a technology’s lifecycle:
A recent Forbes article examines this “Hype Cycle” model concerning the future of data science. It suggests, “We just passed the Peak of Inflated Expectations with data science, and we are about to enter the Trough of Disillusionment…” In the end, the article predicts, “Data science as a degree and as a capability are here to stay, but the job title is not.”
The “trough” indicates that data science is not providing executives with measurable results that can be quantified by a return on investment calculation for solving business problems. Furthermore, “Automation is coming for many tasks data scientists perform, including machine learning. Every major cloud vendor (including Amazon, Microsoft, IBM) has heavily invested in some type of AutoML initiative.”
For large corporations, careful investing in artificial intelligence and machine learning will help keep them competitive. But how can individuals working in data science invest in their future?
A recent Dice article points out that “If you’ve worked in tech for a while, you’ve probably seen a significant number of jobs become obsolete.” They “don’t exactly disappear overnight,” however. “If your job is becoming moribund, you often have time to evolve into a new position.”
Data and computer science majors are used to this kind of career evolution and adaptation. Technology changes so rapidly that they are trained to be lifelong learners who continually update their skill sets. No one sits still for long in data science because the nature of the work is about problem-solving and optimization of the latest data sources with the latest and sharpest tools.
University and Program Name | Learn More |
Boston College:
Master of Science in Applied Economics
|
|
Boston College:
Master of Science in Applied Analytics
|
|
Merrimack College:
Master of Science in Data Science
|
Data science and mathematical science remain fast-growing fields; the US Bureau of Labor Statistics predicts a 31.4 percent increase in jobs between 2020 and 2030.
The work, however, is changing. Data scientists are moving into work a little more closely connected to data engineering. This job title may overtake data science in growth along with artificial intelligence-focused communicators, wranglers, product managers, and architects. As the Forbes article called out, “For complex data engineering tasks, you need five data engineers for every one data scientist.”
Data scientist is third on LinkedIn’s list of the “Top 15 Emerging Jobs in the US,” along with artificial intelligence specialist (#1), robotics engineer (#2), full-stack engineer (#4), and data engineer (#8). This tech family mix generates complicated Venn diagrams of overlapping skills and responsibilities that include core competencies, advanced mathematics and statistics, and data analysis.
The demand for data scientists is great, but how can the specifics for that job remain relevant in a rapidly automating field? As the LinkedIn article “How To Not Become Obsolete as a Data Scientist” explains, to date data scientists have focused on “model building—feature extraction, data cleansing, feature engineering, feature selection, algorithm selection, parameter tuning, model selection, and scoring of datasets.” Because “these processes are now quickly being automated” that are “more accurate and faster than data scientists,” these professionals will need to find new ways to contribute. Fortunately, opportunities should abound.
So instead of asking will data scientists become obsolete, we might ask: how will a data scientist’s job evolve? The answer may just be a shift in job titles, with no change to the already adaptive training a data science master’s degree provides, including knowledge of programming languages, Python, SQL, and Excel. Data scientists will still need to work on programming machine learning algorithms and interpret the findings. Machine learning models are not fully automated—yet.
As the Forbes article predicts, “Some future job titles that may take the place of data scientist include machine learning engineer, data engineer, AI wrangler, AI communicator, AI product manager and AI architect. The only thing that is certain is change, and there are changes coming to data science.” The article suggests that data scientists concerned about becoming obsolete should work on skills that are more challenging to automate, such as creating a machine learning system that “increases key business metrics and produces revenue.”
So, will data scientists become obsolete? Maybe in job title only, if data science grads take the time to sharpen their current skill set with bootcamps and new certifications in data analysis, machine learning, statistical modeling, and deep learning.
Understanding what functions can be easily automated with AI (predictive modeling, data analytics, data visualization, statistical algorithm testing) and what cannot will help underscore the skills that will remain valued and valid for data science teams. After all, statisticians, data analysts, and mathematicians have been the starting job titles for many data scientists, and they’ve evolved alongside new and emerging technologies in real time.
The key is to not let business and technology get ahead of you. Push your adaptive self out of the trough and onto the plateau of productivity. In the coming years, businesses will have to adapt to take advantage of—and not be put out of business by—new technology. The same goes for data scientists who will need to strategize with stakeholders as to how they stay relevant, valuable, and productive in the wake of advances in technology.
Questions or feedback? Email editor@noodle.com
Learning statistical analysis, statistical methods, and statistical theory is a [...]
The human eye blinks an average of 4,200,000 times a [...]
Future health professionals considering the field of eyecare may well [...]
Optometrists have above-average job prospects but face challenges like strict [...]
The US Bureau of Labor Statistics (BLS) forecasts strong sustained [...]
Categorized as: Data Science, Information Technology & Engineering