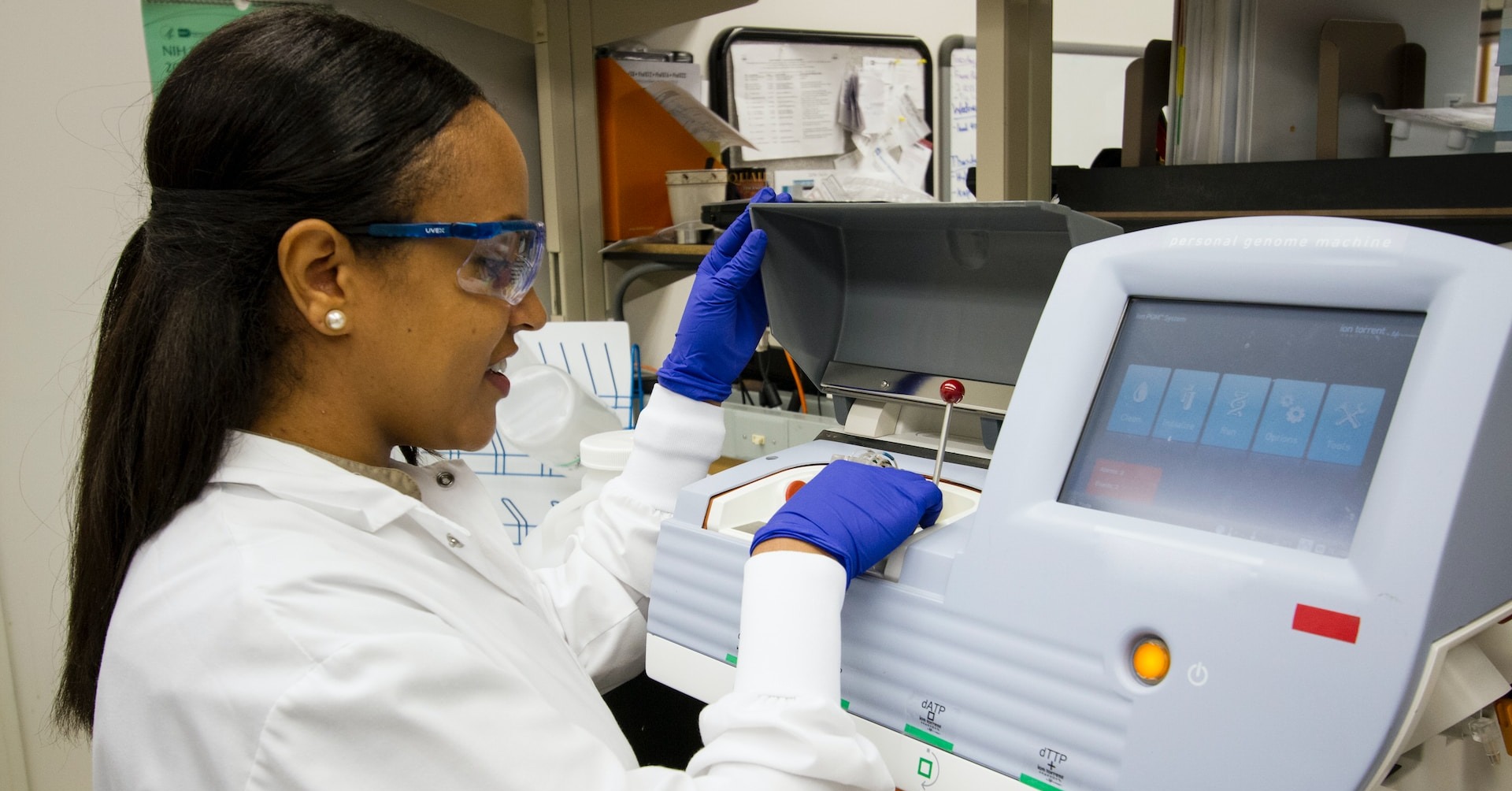
Where Do Epidemiologists Work?
Epidemiologists work in many settings, including hospitals, universities, and federal, [...]
Data science is a branch of computer science recently enjoying a prominent place in the spotlight. Publications call it the sexiest job of the 21st century. Laypeople associate it with cool disciplines like artificial intelligence and machine learning. Business non-quants imagine data science holds the keys to all market mysteries, a Rosetta Stone of consumer information.
There’s still an aura of mystery and glamour surrounding data science that’s unusual in tech fields. And yet, data science is at its core no more than an advanced form of analytics. Oracle sums data science up nicely, calling it a discipline that “combines multiple fields, including statistics, scientific methods, and data analysis to extract value from data.”
Put that way, data science suddenly doesn’t sound very sexy. There’s actually increasing pushback from data scientists tired of seeing data science labeled divination (and data scientists treated like magicians). Data science is a science, but as Alexs Thompson put it in a post on LinkedIn, “Consumers of data science simply throw their hands in the air and use phrases like genius and it’s above my head.”
It’s time to change how we think about what data scientists do and who they are. Becoming one of them—especially now when titles like data scientist have given way to titles like director of machine learning and data engineer—isn’t as simple as making it through a boot camp or taking a few online courses.
In this article, we look at the changing role of data scientist and cover the following:
Data scientists are analytics professionals who explore and find actionable insights in data using various computational tools and techniques to influence decision-making processes. Their work involves collecting, cleaning, organizing, and analyzing massive data sets and developing advanced analytics tools and software designed to solve abstract problems or predict future events. You can find data scientists working to drive business decisions in almost every industry, from commerce and healthcare to logistics and entertainment.
Data scientists are called scientists because of the processes they use in their work. Put simply, data science is the study of information. Like other scientists and academic researchers, data scientists observe rough data, develop testable predictions, create experiments to test those predictions, and draw conclusions from the test results.
Contrary to popular belief, this role didn’t emerge out of nowhere in the early 2000s (that’s when William S. Cleveland published Data Science: An Action Plan for Expanding the Technical Areas of the Field of Statistics in the International Statistical Review). People have conducted statistical analysis with computers since the 1960s; one of the first references to ‘data science’ can be found in Peter Naur’s Concise Survey of Computer Methods, published in 1974. The International Association for Statistical Computing—an organization focused on using computers and statistics to “convert data into information and knowledge”—was formed a few years later.
Businesses and other organizations have been collecting large volumes of data since the 1980s. Still, there were no true data scientists until the technology to work with huge data sets was developed (around the time Cleveland penned his article). When data science tools became widely available, jobs for data scientists rolled out and grew at astounding rates. How fast? Listings for data science jobs increased by 15,000 percent between 2011 and 2012.
Today, jobs for data scientists emerge faster than master’s programs and PhD programs can train students. That doesn’t mean there are necessarily tens of thousands of openings going unfilled, however. The competition for junior data science positions is fiercer than ever before.
University and Program Name | Learn More |
Boston College:
Master of Science in Applied Economics
|
|
Boston College:
Master of Science in Applied Analytics
|
|
Merrimack College:
Master of Science in Data Science
|
Data science is often defined by what data scientists do, but that’s problematic because there are data scientists in different fields doing different things. Increasingly, employers expect data scientists to be domain experts. Some do the sexy work, leveraging data to make self-driving cars safer or to make robots smarter. However, more work for companies in everyday industries like retail, logistics, and finance.
On the other hand, “working data scientists make their daily bread and butter through data collection and data cleaning; building dashboards and reports; data visualization; statistical inference; communicating results to key stakeholders; and convincing decision-makers of their results,” writes Hugo Bowne-Anderson in the Harvard Business Review—regardless of where they work.
We know data scientists gather and organize large amounts of data to solve process and strategy problems in varied fields. Given that, you might assume that job descriptions in data science would be relatively straightforward.
However, that’s not the case. Job postings for data scientists are often frustratingly vague, failing to convey the actual requirements of open positions. They can be misleading or are written by recruiters who don’t have a solid understanding of the technologies and techniques data scientists use.
A general job description for a data science role might read:
Company seeks data scientist to support various teams within our organization by finding opportunities for process optimization in large data sets. Candidate must have experience using multiple data mining and data analysis methods and be comfortable working with a range of departments. Responsibilities include building and implementing predictive models, developing and creating algorithms, and running simulations to improve organizational outcomes and meet short- and long-term departmental and company-wide goals.
It’s tough to nail down what data scientists do because they work on so many types of problems.
The simplest way to sum up the difference between computer scientists and data scientists is that the former solve complex problems using computer design, architecture, and theory while the latter solve problems using large amounts of information. Both computer scientists and data scientists use programming skills and algorithm design. Data scientists employ tools and processes developed by computer scientists. Data science has a much narrower focus than computer science, however.
The work of data scientists and data analysts isn’t that different because data science is essentially advanced analytics. Data analysts use some of the same techniques data scientists use and work with the same kinds of information. Data science is the more technical discipline, however. Data scientists typically do more coding and can leverage technologies like machine learning, artificial intelligence, and algorithms in their work. They’re also more likely to have advanced degrees.
Some employers treat these roles as identical, but they’re not. The typical data scientist has a background in statistics. The typical data engineer has a background in programming. Data scientists use tools to study and solve problems. Data engineering professionals build those tools—or in some cases, tools designed to automate data scientists’ work.
There’s no hard-and-fast dividing line marking the difference between quants and data scientists, which has led to many online debates. While some sources argue that these disciplines differ in critical ways, others say that the data scientist vs. quantitative analyst dispute comes down to semantics. Statisticians who work in tech are data scientists. Statisticians who work in other fields are quantitative analysts. Bolstering the latter viewpoint are the many posters on sites like Reddit and Quora who have been able to apply for both positions without learning any additional technical skills.
Data scientists are programmers, as are software engineers. They sometimes even use the same programming languages, like Python, SQL, Java, Scala, and C++. The difference between these two roles has to do with focus. Data scientists are programmers with a particular goal in mind—to create tools for gathering, organizing, and analyzing data. Software engineers develop applications designed to meet all kinds of needs.
Not all data scientists work in Big Tech, but many do. According to Diffbot’s 2019 State of Data Science, Engineering & AI Report, the seven companies with the largest data science workforces are:
That doesn’t mean tech giants are the only companies employing data scientists. Banks, financial services firms, communications companies, and healthcare networks hire data scientists. There are also data scientists working for nonprofit organizations and local, state, and federal government agencies. The value of data is still unclear in some industries, but that hasn’t stopped companies from collecting vast amounts of information and hiring people to figure out ways to make use of it.
Google employs data scientists to make its products and business processes better. They work with engineers, analysts, and managers to tackle all kinds of challenges and support product development. Hiring standards at Google are high; the company likes to hire PhDs and data scientists with connections to notable people in the field like Geoffrey Hinton and Andrew Ng.
At Facebook, data scientists work closely with the engineering and product teams to suggest metrics-driven changes to products, product roadmaps, and business processes. Working at Facebook—a company known for collecting vast volumes of user information—is a dream come true for many data scientists.
Many data scientists at IBM conduct research and publish on top of internal and external project work. Internal projects involve partnering with teams in software development, market intelligence, fraud intelligence, and chip production. External projects are coordinated by the company’s global consulting department. Data scientists working on external projects solve large-scale problems for Fortune 500 firms.
Amazon’s data scientists can be found across departments, designing forecasting models that predict future customer behavior, developing sophisticated algorithms that automate price setting and recommendations, and using data to streamline logistics. Be aware, however, that most data scientists at Amazon work on just one vertical, becoming domain experts. Many continue to publish, teach, and otherwise engage with the academic community.
The FBI began recruiting data scientists in 2018, which isn’t surprising given that nearly all the national security agencies collect a lot of data. Data scientists who work for the FBI are responsible for identifying mission-critical intelligence in real-time data, using data to reduce risks to agents and the United States, and helping FBI investigators track security violations.
Thinking like a data scientist is something anyone can do with some practice.
Working in data science is much more technical and complex than this, but tackling a problem using these steps can help you understand the discipline’s gist.
All data scientists possess a skill set related to manipulating unstructured data, machine learning, and data visualization.
Above all, however, data scientists need strong communication skills. The insights they derive from data are worthless unless executives and other stakeholders are willing to do something with them. In the Harvard Business Review article linked above, Bowne-Anderson asked consultant Jonathan Nolis, “Which skill is more important for a data scientist: the ability to use the most sophisticated deep learning models, or the ability to make good PowerPoint slides?” Nolis made a case for the latter skills (communication) being more important.
Smart data scientists put together resumes that target employers, not other data scientists. When putting together your resume, your goal shouldn’t be to show off your data mining and programming chops but to prove to hiring managers (who may not have a tech background) how much value you can generate with your skills.
Show, don’t tell. Use concrete metrics whenever possible, but make sure those metrics are interesting to employers. Did you generate revenue? Triple conversions? Boost market share by 15 percent? Concrete metrics make prospective employers think about how you will boost their bottom line.
A good rule of thumb is to share information on your resume in this order:
These are some of the most common technical questions interviewers ask candidates interviewing for data science positions:
There’s no such thing as the ideal data scientist cover letter. Every cover letter you send should be tailored to the job you’re hoping to get.
That doesn’t mean you have to write each cover letter from scratch. You can create a template for open positions and tweak it to ensure it’s in line with what a given employer is looking for. You should always state why you’d be a great fit and why you’d like to work for the company, demonstrate how your experience harmonizes with the role’s responsibilities, and thank the employer for their consideration.
Data science careers can be quite lucrative. Experienced data scientists earn salaries in the low six-figure range. In managerial roles—like director of data science— data scientists can earn close to $200,000—or even more at big tech firms.
The average data scientist earns about $123,000, though it’s important to consider that figures like that are calculated using salary data from both the lowest-paid and highest-paid data scientists. Salaries in data science are determined by factors like work experience, highest level of education, location, and company type and size. A data scientist working for Google, for example, will almost certainly earn more than one working for a regional nonprofit.
Entry-level data scientists, according to ZipRecruiter, earn around $165,000, which is higher than entry-level salaries in other industries. However, keep in mind that many “junior” data scientists have master’s degrees and mid-level analytics experience.
According to the most recent Robert Half Technology Salary Guide, the median national salary for data scientist roles in 2024 is $144,250. That’s good, though it’s not much more than data scientists were earning in 2016 and 2017. Chances are that data science salaries will stay high, but the increasing number of data scientists plus new automation techniques may keep those salaries relatively level.
The answer to this question depends on whether you enjoy finding needles in haystacks—or programming computers to find needles in haystacks—and how flexible you are. Because data science has applications in so many industries, you can’t always predict what field you’ll end up in. You might work for a retailer, pharmaceutical company, or energy company, but your job will probably involve using the information to make money for your employer in all three cases.
Nearly all data scientists have bachelor’s degrees. 90 percent hold advanced degrees. Data science resource KD Nuggets found that 88 percent of data scientists have at least a master’s degree and 46 percent have PhDs, though not necessarily in data science.
Many data scientists come from predictable undergraduate backgrounds like computer science and math, but others launch their early careers with bachelor’s degrees in physics and biology. At the master’s level, some data scientists earn degrees in data science, but others study computer science, math, statistics, or engineering. Many data scientists learn to do what they do outside the classroom.
In some disciplines, you can jump into a graduate program with no experience. Data science isn’t one of them. Top data science master’s programs attract students with professional experience in data analytics, business intelligence, computer engineering, or statistical mathematics. Students enroll to sharpen their skills, and programs of study in the best data science master’s programs tend to be concentration-based.
Some schools, however, do have generalist data science master’s programs. The curriculum of the University of Virginia’s online Master of Science in Data Science program, for instance, is made up largely of foundational courses like:
Data science doctorate programs are often much smaller than computer science programs. It’s not unusual for them to be highly competitive when it comes to admission. Top programs look for applicants with extensive work or research experience and advanced degrees in related fields.
The pros of becoming a data scientist are obvious and compelling. Data science is still seen as one of the hotter branches of computer science, and data scientists are in demand. Salaries are high, data scientists advance quickly, and the job market is robust because so many industries now generate massive quantities of potentially useful data. That makes it relatively easy for data scientists to move between fields. Finding actionable insights in data is also interesting work for those with an affinity for it. Jobs in data science are hailed by many sources as some of the best jobs in America.
There are cons to consider, too, when exploring this career path. There’s no industry-standard definition employers can refer to when hiring data scientists, so data science jobs can vary widely from company to company. Data science also suffers from the same diversity issues that plague other areas of computing. Women and minorities can face an uphill battle when it comes to advancement in the field. And the data science landscape is evolving rapidly, so this isn’t a get-your-degree-and-coast type of job. When change happens in data science, it’s dramatic, and professionals often have to scramble to get back up to speed.
You may have wondered whether you can become a data scientist without a degree. The answer is complicated. Yes, you probably need a degree to advance past a certain point in this field, given how common master’s degrees and doctorates are in the data science world.
However, that doesn’t mean that you can’t learn data science outside a degree program or strengthen your skills without going back to school.
Data scientists have to be lifelong learners, regardless of whether they pursue additional degrees or data science certifications because this discipline never stops evolving. As technology changes, data science will change—and data scientists with it. There will always be new certifications to earn, new tools to learn, and new techniques to explore. When you become a data scientist, your training never ends.
(Last Updated on June 3, 2024)
Questions or feedback? Email editor@noodle.com
Epidemiologists work in many settings, including hospitals, universities, and federal, [...]
According to the Bureau of Labor Statistics, artificial intelligence and [...]
Modern business intelligence is all about data, which is why [...]
A master's in homeland security prepares students for careers in [...]
Genetic counseling is a career that combines science and sensitivity,especially [...]
Categorized as: Data Science, Information Technology & Engineering