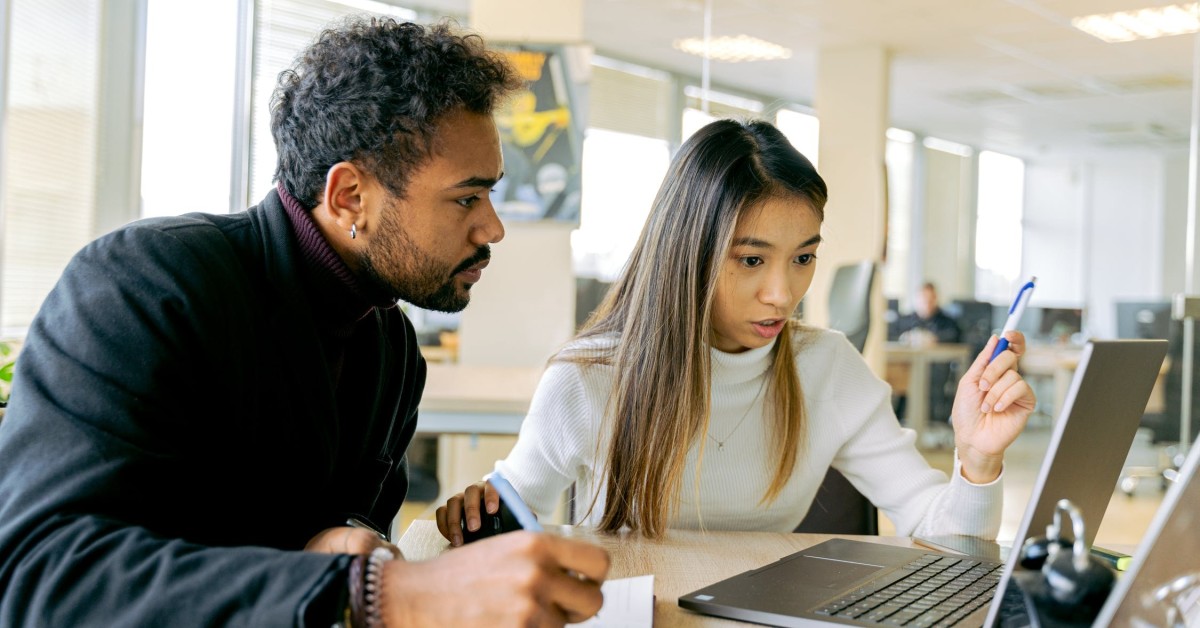
Artificial Intelligence Master's: What Are the Admissions Requirements?
You're ready to boost your AI career and apply for [...]
Most of the data scientists in the world, if LinkedIn co-founder Allen Blue is to be believed, are already gainfully employed. “There are very few data scientists out there passing out their resumes,” he told the Knowledge at Wharton blog, adding that data science and machine learning jobs represent five of the top 15 fastest-growing jobs in America. The Bureau of Labor Statistics confirms that, setting the job market growth rate between 2021 and 2031 for data scientists in the United States at 36 percent, seven times faster than the rate for the overall job market.
The takeaway? There are more data science jobs than there are data scientists to fill them. If you’re looking for job security (and who isn’t), you can find it in this highly technical, highly specialized field.
Just because demand for data scientists is high doesn’t mean you should become one, however. If you’re researching careers in data science because you’re operating under the assumption that it’s an easy path to big money, you need to take another look at the skill set data scientists have and what data scientists actually do. Data scientists need to be experts in math, statistics, programming, communication, and problem-solving. But more importantly, they need to love data. Harvard Business Review called data scientist the sexiest job of the 21st century, but the reality is that this is a job for hardcore numbers nerds.
Massachusetts Institute of Technology graduate Kay Aull put it like this in a Quora thread about choosing a data science career: “There’s data everywhere. Have you ever said ‘Hmm, I wonder…’ and then lost hours of your life trying to kludge together an analysis? If so, you might be the right kind of weirdo for data science. If you just want to learn a few buzzwords and then stroll into a six-figure job, there are other fields better suited for that.”
Let’s assume you’re not here for the buzzwords or because you want us to confirm that data scientists make good money (they do). In this article, we’re going to dive deep into the question “What does a data scientist do?” and cover the following ground:
Figuring out what data scientists do isn’t always easy. As you research the question, you’ll probably come across answers like this: Data scientists develop predictive and prescriptive algorithms built on clean data sets. Unless you’re already a data scientist, that explanation may be head-scratchily vague. This description of what data scientists do is easier to understand: Data scientists gather and organize large amounts of data to solve process and strategy problems in business and in other enterprises.
We can even break it down further. Data scientists explore data with powerful tools (which they sometimes design themselves) to discover meaning. It’s still a vague answer, but purposefully so, because data science is useful across industries. Whether a data scientist works in pharmaceuticals or finance doesn’t matter. They’re still using huge sets of information to solve abstract problems or predict future events.
Here’s an example of data science in action: An insurance company wants to reduce costs by catching a specific cancer earlier when treatment is less expensive. It uses analysis of data related to patient screenings, treatments, and outcomes to determine which subscribers will benefit most from which screenings.
Here’s another: An e-commerce company wants to increase the amount customers spend in a single shopping trip. It uses analysis of past customer behavior to generate product recommendations related to what’s already in a customer’s virtual shopping cart.
Data scientists do more than just make money and save money for corporations, however. Nonprofit organizations, academic institutions, and governments all use data science to optimize processes, answer strategy questions, and identify trends. Sometimes data scientists are given a complex problem, and they have to decide which information they’ll need to solve it. Sometimes they’re given information and asked to extract as much meaning from it as possible. In both cases, math, statistics, and programming will come into play.
University and Program Name | Learn More |
Boston College:
Master of Science in Applied Economics
|
|
Boston College:
Master of Science in Applied Analytics
|
|
Merrimack College:
Master of Science in Data Science
|
Yes and no. Data analysts work with information in many of the same ways data scientists do. And the work of data scientists and data analysts can overlap. The biggest differences between these two professionals can be found in the base level of technical expertise each possesses. Data science is a lot more technical and hands-on, and data scientists are much more likely to have coding skills, machine learning expertise, an understanding of artificial intelligence, experience with predictive analytics, and mathematical modeling expertise. They’re also more likely to have advanced degrees. In fact, data analysts sometimes advance into data scientist positions after earning a master’s degree in data analytics.
To do what they do with large data sets, data scientists need to be proficient in:
Getting experience before working in a data scientist role or even a degree is pretty easy nowadays. Just look at what data scientists do and try to do it yourself. There are lots of free data sets you play around with—Kaggle has 19,000 public datasets—and plenty of free data manipulation tools and programming tutorials. You can learn so much about what data scientists do (and whether you want to devote your career to doing those things) by spending your free time visualizing data and building machine learning models.
You may also land a job more quickly if you build up a portfolio of personal projects that highlight your technical skills and knack for communicating results in a way non-techy folks can understand.
The answer to this question isn’t particularly straightforward. Unsurprisingly, most data scientists have both bachelor’s degrees and advanced degrees (47 percent hold master’s degrees and 47 percent hold doctorate degrees), and most specialize in computer science, math, statistics, or engineering for their entire academic careers. But data scientists also come from undergraduate backgrounds like electrical engineering, physics, and biology, and many data scientists are at least partially self-taught.
When aspiring data scientists are ready to enroll in a master’s degree or doctoral program, they typically opt to study subjects like data analytics, data engineering, applied statistics, and business intelligence because there still aren’t that many schools offering data science degrees. Some high-profile colleges and universities offer master’s degrees in data science master; they include Harvard University, Columbia University, and Tufts University. You’ll also find relevant graduate degree programs at:
Data science training doesn’t end at graduation because earning an advanced degree won’t turn you into a data scientist. The reason there are so many self-taught data scientists is that degree programs don’t necessarily cover the ins and outs of things like Hadoop data lakes or Big Data querying—and if they do, the information they give students may be out of date by the time those students get their diplomas. New data science techniques and tools are being developed all the time. One thing you’ll never stop doing if you become a data scientist is learning.
Take a guess, and you might say that big tech companies like IBM, Amazon, and Microsoft are employing the most data scientists. You wouldn’t be wrong. Diffbot’s 2019 State of Data Science, Engineering & AI Report identified the companies with the largest data-related workforces:
But the tech giants aren’t the only companies that can see value in what data scientists do. Financial companies like Fidelity Investments and Bank of America hire data scientists to interpret data. So do pharmaceutical companies like Bristol-Myers Squibb. Communications companies, retailers like Target and PetSmart, hospitals, marketing firms, and even fashion houses find applications for data science. Data scientists can be found doing what they do just about everywhere because data science can answer so many kinds of questions—and boost profits.
The list of tools data scientists use is long, but it’s important to remember that computer technology changes rapidly. By the time you read this article, there may be newer, more effective data science tools that are making it easier to clean, sort, and analyze information. Right now, data scientists rely on tools like:
Data science is about more than just using tools, however. Data scientists also have to know when to use different tools. Small data sets can be cleaned and analyzed using Excel, for example. Given how many sophisticated data science tools there are, it’s surprisingly useful. When time is of the essence and there’s a lot of data, R language is the best choice.
A lot, if you know what you’re doing and have an advanced quantitative degree. The Bureau of Labor Statistics sets median data scientist annual pay at just over $100,000. Those in top-paying states fare substantially better:
Top-paying sectors include:
(Updated on July 3, 2024)
Questions or feedback? Email editor@noodle.com
You're ready to boost your AI career and apply for [...]
Most data science professionals have a PhD, but do you [...]
Categorized as: Data Science, Information Technology & Engineering