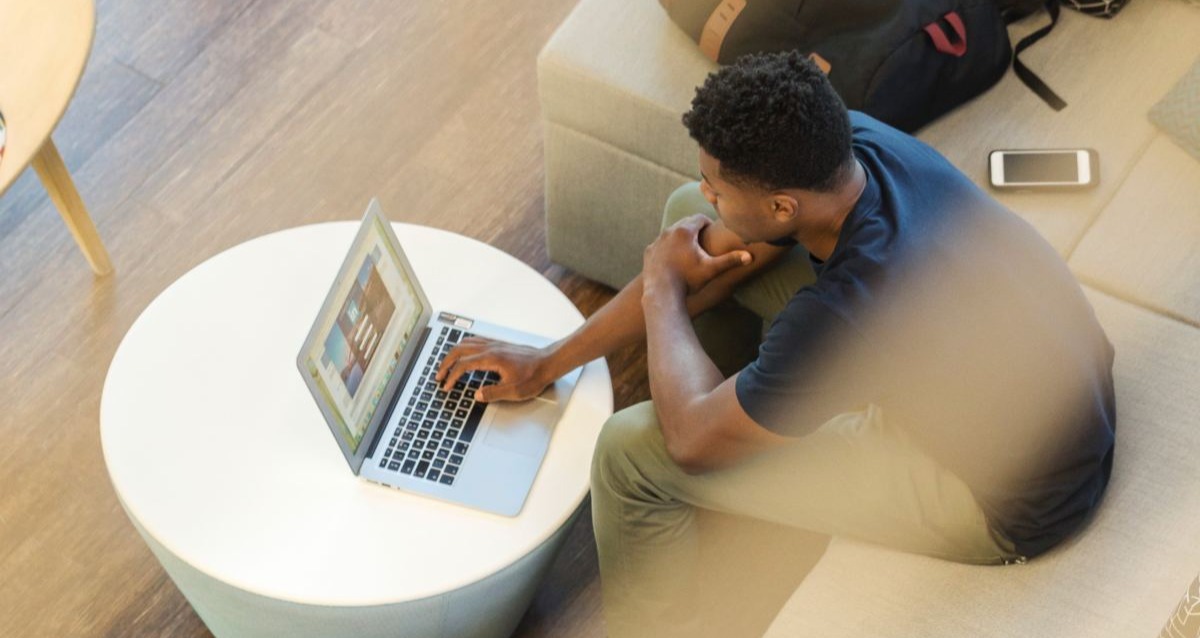
Machine Learning vs Artificial Intelligence: What's the Difference?
According to the Bureau of Labor Statistics, artificial intelligence and [...]
Though the term data scientist first appeared in the modern lexicon around 2008, data science itself has roots all the way back in John Tukey’s 1962 paper “The Future of Data Analysis”. In it, Tukey foretold the merger of data analysis and computer science.
From the 1960s to the 1990s, statisticians primarily published papers and conducted research. In 1977, The International Association for Statistical Computing (IASC) started striving “to convert data into information and knowledge” through expert research in statistics and computer science. At the time, data science was primarily theoretical, with few real-world applications.
Fast forward to modern times. An astounding 2.5 quintillion data bites were produced daily in 2020. New technology, practices, and problem-solving techniques mean that number will only keep growing.
Big data helps companies of all sizes, from small businesses to Amazon. Advanced machine learning algorithms can now glean insights into user data on a massive scale. Even sports franchises employ data science to identify the smallest areas of player optimization on the field.
There may come a day when data scientist is no longer one of the best jobs, but that appears to be a long way off. For now, data science is a field with many opportunities, a surprising number of them on the cutting edge.
So, when did data science start? This article looks into that and also discusses how to land one of the many high-paying data science jobs. It covers:
Modern data science emerged in the 1990s and early 2000s, but technology and practices had not yet caught up to needs. Many companies collected consumer data to inform marketing campaigns—often without much success—back in 1994. The term data mining became popular shortly afterward, and many professionals recognized the need to comb through large data sets to find information, though they still lacked the tools to do so.
Data science entered the modern era along with the development of efficient methods for solving business problems and, of course. increased computing power. Professionals learned to read metrics and utilize data sources for decision-making purposes. In 2005, Thomas H. Davenport’s paper “Competing on Analytics” discussed how “companies are beginning to employ statistical and quantitative analysis and predictive modeling as primary elements of competition.” Decades-old concepts became building blocks for innovation. Data analysts started to present information using data visualization techniques that other stakeholders in a company could use as business intelligence.
The real explosion began in 2009 when the chief economist for Google, Hal Varian, predicted that statistician jobs would be “sexy” in the next decade. Later that year, Nathan Yau said Varian argued that data scientists, as he called them, were already popular. The 2010s saw an explosion of data science, and much of the progress is yet to come. Once fledgling subsets of data science—such as artificial intelligence, deep learning, and machine learning—now have viable uses. Cars are starting to drive themselves; one can only imagine what the next developments will bring.
Data scientists can have different skill sets, depending on their niche. Generally, specialization leads to better jobs. Top data science positions and their average national pay according to Glassdoor, include:
Data architects are responsible for building and operating database systems. To succeed, they collect data using machine learning models to make it understandable through visualization tools. However, architects do not conduct analysis; rather, they build the systems that facilitate analysis. The average annual salary for this position is more than $150,000 with top earners collecting around $330,000.
Data engineers perform many of the same data management tasks as data architects, including constructing and maintaining databases and creating pipelines to transport information. Data engineers may work under a data architect, as they’re typically less experienced. They earn nearly $115,000 per year, and the top of the pay range is $384,000.
Data scientists leverage data analytics for decision-making, differentiating the role from positions that focus on data collection. The top data scientists are among the best-paid in the field, earning nearly $675,000 per year. However, the average data scientist earns a considerably lower, but still excellent, salary of $122,00 per year.
Enterprise architecture is the practice of creating IT systems for an organization. Enterprise architects typically occupy managerial roles, meaning they don’t participate in the coding but rather direct a team. In this managerial position, they collaborate with executives to create plans and adapt to changes in the organizations. Enterprise architects earn nearly $165,000on average, with top earners making $855,000.
This role requires an excellent machine learning skill set, as well as competency with modeling and mining data. As a machine learning engineer, you may work with data scientists to interpret your findings. These professionals earn almost $123,000 on average—top professionals can make nearly $400,000.
Keep in mind that all of the salaries reflect national averages. You often can earn above the average working in a tech hub like New York or California. Aside from location, experience, industry, and education play a role in your earnings. Finally, data science is a perk-filled field. A start-up may not pay as much as Microsoft (at first) but may offer potentially valuable stock options and other incentives. If the business takes off, you may be in a better position than if you had pursued a position at an established corporation.
University and Program Name | Learn More |
Boston College:
Master of Science in Applied Economics
|
|
Boston College:
Master of Science in Applied Analytics
|
|
Merrimack College:
Master of Science in Data Science
|
Most working data science professionals (42 percent) have a master’s degree as their highest level of education. PhDs are the next-most common (at 38 percent). All of which suggests that a bachelor’s degree can only take you so far in this field.
Bootcamps and self-taught success stories may tempt you, but earning a master’s in data science is one of the best ways to solidify your career. Recruiters struggle to find qualified candidates for the high-level positions mentioned above. Most applicants just don’t have the required skills.
You can reasonably expect to earn a master’s degree in two years, though your grad program may be longer or shorter depending on whether you choose a part-time or accelerated track.
Most data science programs look for applicants with experience in data or a related field. Those with a STEM degree have improved chances of being admitted. It’s possible to be accepted to a program without a relevant background, but you may need to complete prerequisite coursework or self-study.
In addition to your resume, you can expect to submit the standard graduate school admissions requirements like a personal essay, standardized test scores (unless your program is test-optional), letters of recommendation, and prior transcripts (some programs require a GPA of 3.0 or higher). You may need to sit for an interview.
Most data science programs include core coursework in advance mathematics (including statistics and algorithms), programming languages (Python, Java, C++, etc.), and data analysis (including visualization).
You’ll also be able to specialize during a data science degree program, often through a structured track, including a capstone or thesis. Or, you may complete electives in the area of your choice. Common specializations include big data, cybersecurity, bioinformatics, machine learning, and artificial intelligence.
Well-regarded data science degree programs can be found at:
Questions or feedback? Email editor@noodle.com
According to the Bureau of Labor Statistics, artificial intelligence and [...]
A master's in homeland security prepares students for careers in [...]
Cyber security analysts earn around $105,000 per year on average. [...]
Genetic counseling is a career that combines science and sensitivity,especially [...]
If you're hoping to submit your business school applications anytime [...]
Categorized as: Data Science, Information Technology & Engineering