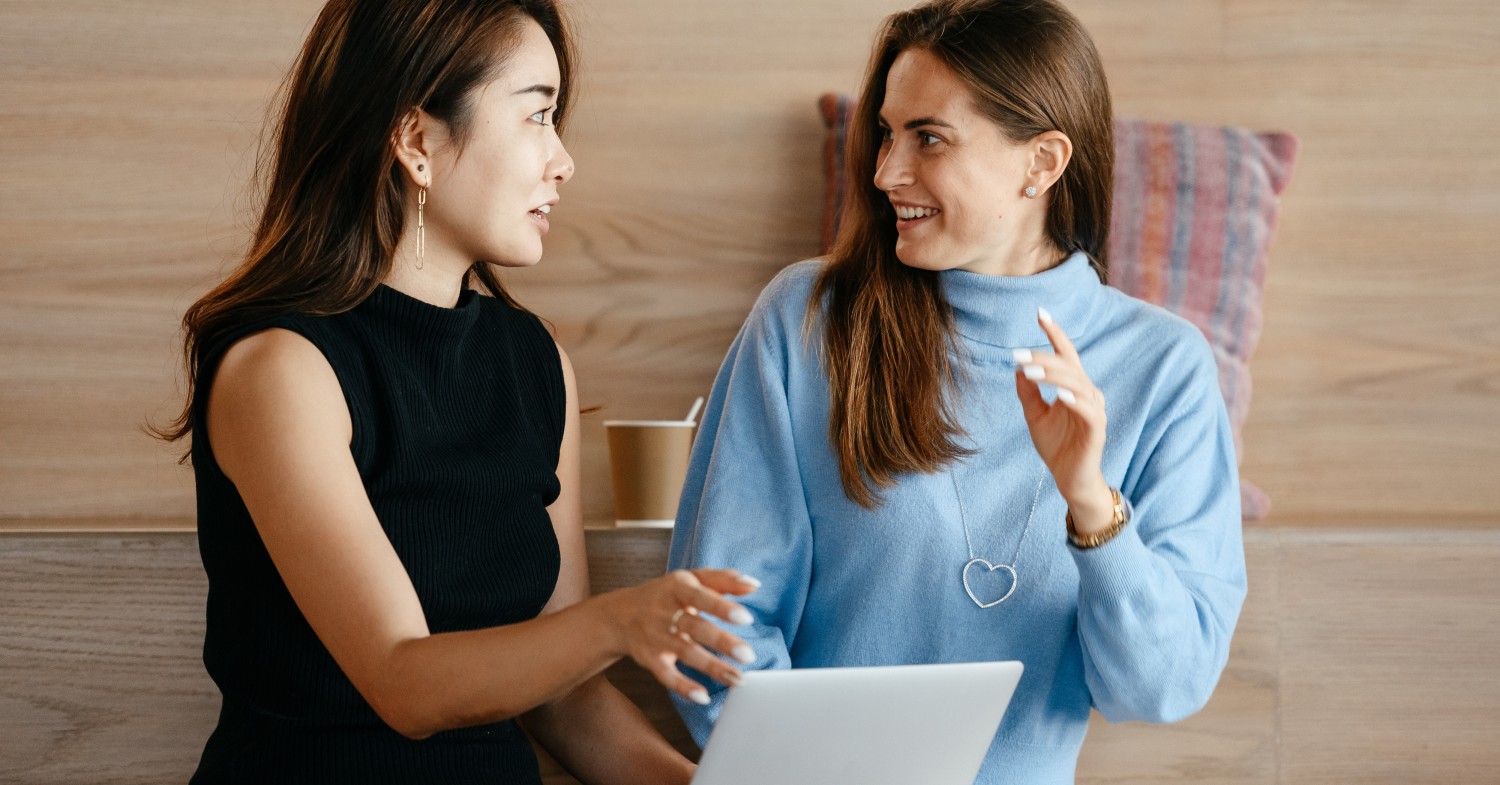
What Does a Director of Communications Do?
A team of PR professionals shapes a company's voice, brand, [...]
For any structure to provide a reliable framework for growth and development, it must begin with a solid foundation. This is true for any type of construct: social, psychological, metaphorical, or physical. While there are lots of visual models that help demonstrate these structural levels, the pyramid—with its strong base supporting its subsequent layers of rising heights—is perhaps the most appealing.
This is the shape chosen to represent Abraham Maslow’s hierarchy of needs, first introduced in his 1943 paper titled “A Theory of Human Motivation”. While Maslow himself didn’t use the pyramid to introduce his idea to the larger study of human developmental psychology, his theory is easily adapted to its shape and structure, and is frequently represented in this fashion.
Maslow’s hierarchy framework rests on the theory that humans have foundational needs which must be met first before they will seek to move to the next level of development. The bottom layers and base of the pyramid contain the deficiency needs, beginning with and building upward from: physiological (food, water, warmth, rest), safety, belonging and love (intimate relationships and friends), and self-actualization/esteem (achieving full potential).
Maslow’s argues that when the first layers of basic needs are not met, people are less likely to be motivated toward transcendence and self-actualization, which occupy the very top of the pyramid. With the basic physiological, safety, and belonging needs settled and satisfied—step by step- it is then possible for a person to work on the higher-level growth needs: cognitive, aesthetic, self-actualization, and transcendence.
The pyramid’s visual shape and the framework of Maslow’s hierarchy both easily lend themselves to the structure of data science and the functionality of its use in business. Monica Rogati uses this model in outlining “The AI Hierarchy of Needs,” substituting Maslow’s human needs with the building blocks of data science. She explains: “Think of AI as the top of a pyramid of needs. Yes, self-actualization (AI) is great, but you first need food, water, and shelter (data literacy, collection, and infrastructure).” If artificial intelligence is at the top of the data science hierarchy of needs, what makes up the foundational layers of the data science pyramid?
Rogati argues that companies must prepare for data collection and require a reliable and effective framework in place to benefit from the information the company collects. It’s just not possible to begin by building machine learning algorithms without data structure in place. “More often than not, companies are not ready for AI. Maybe they hired their first data scientist to less-than-stellar outcomes, or maybe data literacy is not central to their culture. But the most common scenario is that they have not yet built the infrastructure to implement (and reap the benefits of) the most basic data science algorithms and operations, much less machine learning.”
To follow Maslow’s model and work from the foundation up, we need to start with data collection: What data do you need, and what is available to you? This is a critical stage for any data-driven enterprise. It provides the footing for the loftier goals of the company.
According to data science consultant Matthew Renze, “The most basic need of a data-driven organization is the need to collect data. This starts with basic data collection activities like recording transactions, logging errors, and digitizing analog data.” Before moving to the next pyramid level, organizations need to look at the data coming through sensors and the ways relevant user interactions are being logged to create a solid dataset.
With accurate, reliable, and complete data collection, an organization is ready to advance to securing movement, organization, and storage—or monitoring how the data flows through the system. Accessible data is useful data, and this is the stage to test data sources and sensors. Basic, unstructured organization begins here, with further structure and storage development to follow.
The next level of the data science hierarchy of needs pyramid involves exploration and data analysis through anomaly detection and data cleaning. This is an important step in preparation for a more robust organization of data at the next level. If the results are less than stellar, it may be time to check the first-level foundation and refocus on collection methods.
Once data is reliably explored and organized, metrics can be defined and analytics can begin. Data storage becomes more important as the company matures, which may lead to more robust solutions.
As we near the uppermost level, the pyramid has achieved analytics, metrics, and training data. Now it’s time to test, learn, and optimize data usage. Are you ready for machine learning? According to Monica Rogati: “Maybe, if you’re trying to internally predict churn; no, if the result is going to be customer-facing. We need to have a (however primitive) A/B testing or experimentation framework in place, so we can deploy incrementally to avoid disasters and get a rough estimate of the effects of the changes before they affect everybody.”
This is it—the place where the strength of the pyramid proves itself. With cleaned and organized data, proper instrumentation, dashboards, labels, and good measurement, all is in place to move into artificial intelligence and deep learning. You can begin to experiment and, because you have lots of good data, scale up the use of machine learning models. This level also allows for automation and predictive analytics born out of big data.
The importance of this structure cannot be overstated. In early startups and even in large, existing corporations there is a need to begin at the beginning and secure the foundation to support the larger goal. Those first levels may need to be revisited and altered as a company grows and evolves.
In their article in Harvard Business Review, “If Your Company Isn’t Good at Analytics, It’s Not Ready for AI”, Nick Harrison and Deborah O’Neil outline the need for companies to have a strong framework when analyzing less structured data.
“Artificial intelligence systems make a huge difference when unstructured data such as social media, call center notes, images, or open-ended surveys are also required to reach a judgment…” They note that fund managers well-versed in data analytics “are predicting with greater accuracy how stocks will perform by applying AI to data sets involving everything from weather data to counting cars in different locations to analyzing supply chains.”
A solid foundation is important for building any business, and with a clear visual outline of the structure needed, it is easy to comprehend the layered process. It’s also easy to see the importance of not skipping a step. Investing the necessary time, money, and energy in the preparation of data will allow for the most accurate and useful outcomes in business from the pinnacle workings of AI.
Questions or feedback? Email editor@noodle.com
A team of PR professionals shapes a company's voice, brand, [...]
Public relations officers manage a company's or client's public profile [...]
There's a good chance that earning a network engineering master's [...]
Whether you're bored at your current job or simply ready [...]
A master's in network engineering is a relatively new degree, [...]
Categorized as: Data Science, Information Technology & Engineering